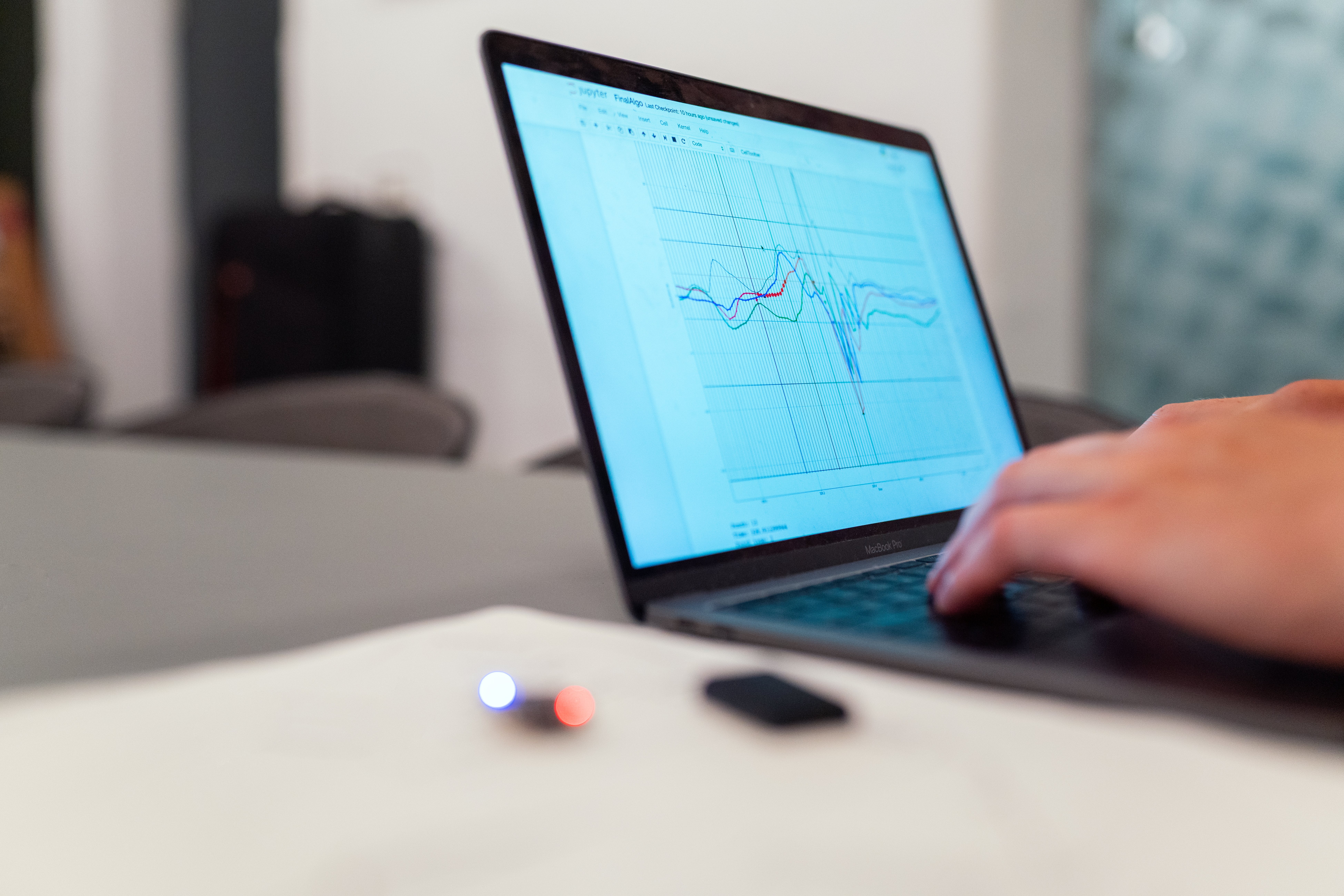
Job Profile
Data Scientist
Data Scientists analyze and evaluate unstructured data. On this basis, they make predictions and decisions that determine the course of a project.
Data Scientist Job Profile
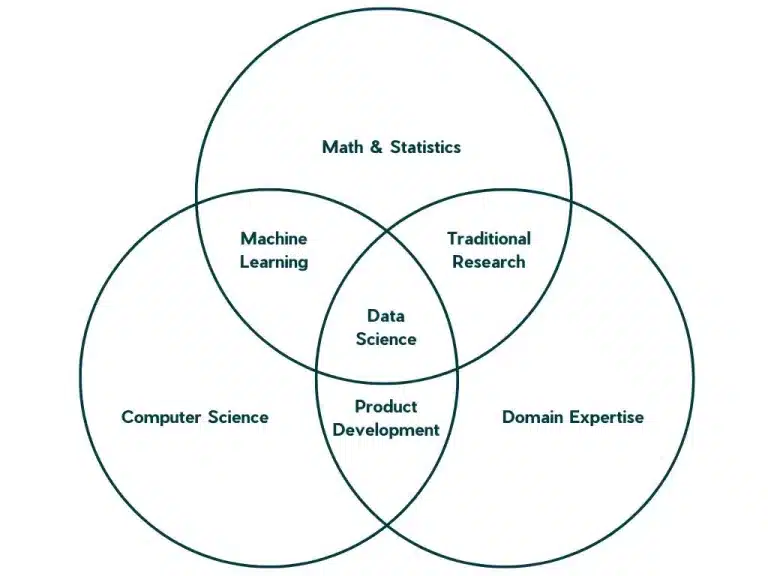
What Is the Salary of a Data Scientist?
In Germany, the average salary for a data scientist is roughly 5,960 EUR per month. The lowest salary is 2,740 EUR, and the highest is 9,470 EUR (highest).
With housing, transportation, and other benefits included, this is the typical monthly wage. Based on experience, abilities, gender, or region, data scientist compensation might vary greatly. The wage is primarily influenced by the level of experience. Naturally, your salary will increase as you get more experience. These are the results of our analysis of Data Scientist salaries according to level of experience.
The average monthly salary for a data scientist with less two years of experience is 3,110 EUR.
A person with two to five years of experience may expect to make 4,150 EUR per month, which is 34% higher than someone with less than two years of experience.
Moving forward, someone with five to 10 years of experience earns 6,140 EUR per month, which is 48% more than someone with only two to five years of experience.
A Data Scientist’s compensation similar to 7,480 EUR per month, or 22% more than someone with five to 10 years of experience, is also paid for experience spanning anywhere between ten and fifteen years.
A person with fifteen to twenty years of experience can expect to make 8,160 EUR a month, which is 9% higher than someone with 10 to fifteen years of experience.
The wage for workers with more than twenty years of experience in their field is 8,830 EUR per month, which is 8% more than the salary for workers with fifteen to twenty years.
What Are the Tasks of a Data Scientist?
To automate data gathering and storage processes, data scientists use coding and other programming techniques. They might collaborate closely with internal business divisions or even design a mechanism for storing gathered data in their databases. Data Scientists assist businesses by offering them insightful advice on how to enhance daily operations.
Duties and responsibilities
Data Scientists use their programming expertise to create automated systems that assist businesses in streamlining business processes. They are responsible for gathering raw data from various sources, analyzing outcomes, or designing new studies, and converting it into an appropriate format for analysis. They must also collaborate closely with other departments and decide what data should go where and how much information is required, all while following the rules established by law or regulation.
The link between business and data defines a good Data Scientist. They must be able to visualize their results and possess a strong technical understanding of how to convey complex facts in an understandable manner. A skilled data scientist will also be proficient in writing, analysis, and problem-solving.
A Data Scientist often collaborates with a group of other data scientists inside a company or organization to evaluate various types of data. They might inform superiors, like a Lead Data Scientist, of their findings and progress.
What Skills Does a Data Scientist Need?
- Experience in data mining
- Knowledge of R, SQL, and Python; familiarity with Scala, Java or C++ is an asset
- Experience using business intelligence tools (e.g., Tableau) and data frameworks (e.g., Hadoop)
- Proficient math skills (e.g., statistics, algebra)
- Bachelor of Science/Bachelor of Arts in Computer Science, Engineering or similar
- Problem-solving mind
- Excellent communication and presentation skills
- Understanding of machine-learning and operations research
- Graduate degree in Data Science or another quantitative field is recommended

Find qualified Data Scientists.
What Is the Difference Between a Data Scientist and a Machine Learning Engineer?
Data scientists and machine learning engineers both have hands-on responsibilities, yet their daily activities are very different from one another.
The builders are often referred to as data scientists. They must identify and comprehend certain business issues, feature engineer models, construct, pick, and fine-tune them, and then produce insights to share with stakeholders. On the other hand, ML engineers are primarily concerned with taking these models and assisting in their scale out into production while assuring compliance with business SLAs. They are in charge of incorporating models into a company’s workflow and are often more involved in maintenance and monitoring than creation.
Machine Learning Engineers are responsible for executing a risk mitigation approach and making sure the models function properly while pursuing the intended objective, especially in enterprise settings.
Imagine that Data Sience was a brand-new housing development. Data scientists would be in charge of designing specialized blueprints and floorplans and selling them to builders before production, much like how they “sell” their model ideas to leadership. The properties would be listed on the market, the property would be maintained, and all the inhabitants would be kept happy by Machine Learning Engineers.
What Is the Role of Big Data In Data Science?
Big Data is basically a specific application of Data Science whenever the data sets are very large and handling them involves solving logistical difficulties. Efficiency in gathering, storing, extracting, processing, and interpreting data from these massive data sets is of utmost importance.
Due to physical and/or technical limitations, processing and analysis of these enormous data sets are frequently not practicable or possible. Therefore, specialized methods and equipment (such as software, algorithms, parallel programming, etc.) are needed.<
These vast data sets, specialized methods, and unique technologies are together referred to as Big Data. In order to do broad data analysis, identify trends, or develop prediction models, it is frequently applied to enormous data sets.
The so-called Three Vs (3Vs) paradigm is a key element of big data. This model illustrates how the volume, variety, and velocity of big data relate to its characteristics and difficulties. Veracity is a fourth “V” used by organizations like IBM, and Wikipedia also mentions variability.
Big data generally seeks to address the issue of handling vast quantities of data of various quality, frequently of numerous distinct types, that are being collected and processed at times at extremely fast (real-time) rates. Certainly not a simple task!
Therefore, in order to address a problem, an entity (person, corporation, etc.) must use specialized hardware, software, processing techniques, visualization, and database technologies. This is what is meant by the term “Big Data,” which is a relative word that refers to massive data sets.
Find Qualified Freelance-Experts.
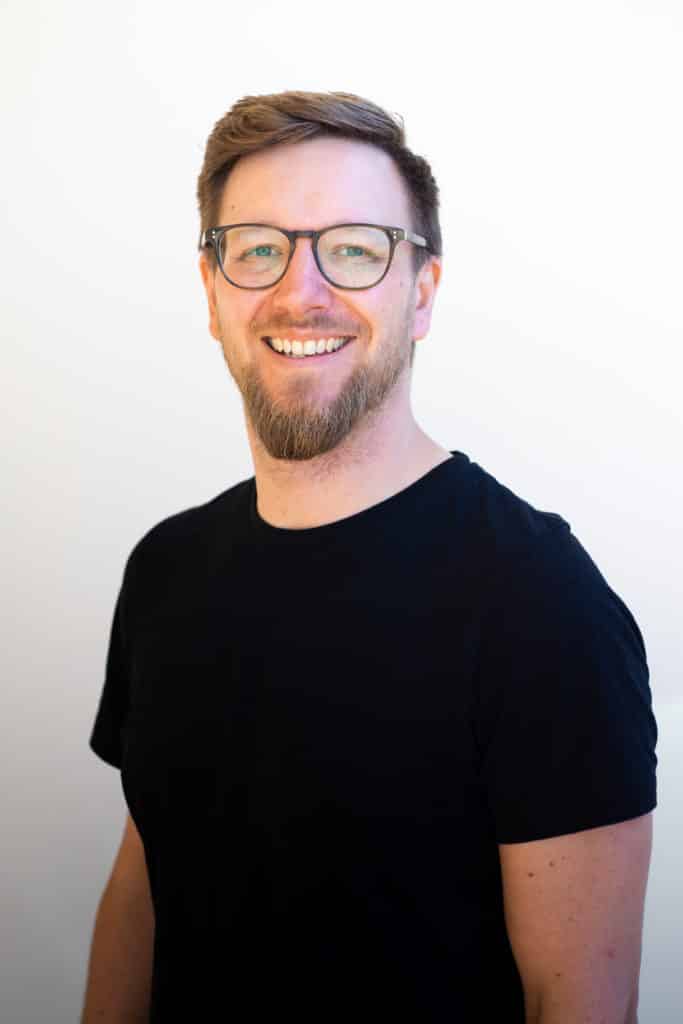
Your Contact Person
Sören Elser
Co-founder of ElevateX GmbH and your contact for the strategic use of freelancers.