Welcome to the exciting topic of AIOps! In this blogpost, we explore the fascinating world of Artificial Intelligence for IT Operations and how this innovative technology optimizes IT infrastructures through artificial intelligence and machine learning.
What Is AIOps?
AIOps is the abbreviation for Artificial Intelligence for IT Operations. The term refers to the use of Artificial Intelligence (AI) and Machine Learning (ML) in the utilization, management, and monitoring of IT infrastructures and services. It was coined by Gartner, a US-based IT research and consulting firm, in 2017. The approach is defined as increasing the efficiency of data collection, analysis, automated processing, and evaluation through the integration of AI and ML. Further information about the Gartner research portfolio can be found here.
The implementation of AIOps enables IT infrastructures to analyze ever-growing volumes of data, detect anomalies, respond faster to disruptions, take preventive measures, and overall, make IT operations more agile. The successful implementation of such complex structures requires skilled IT professionals, as presented on our webpage.
What Does AIOps Have To Do With Artificial Intelligence?
AIOps utilizes the structures and mathematical algorithms of AI, Big Data, and Machine Learning to automate operational processes, optimize and monitor IT systems. The data collected by IT systems is organized, managed, and analyzed without human intervention. Reactions can happen immediately and in real-time. By employing AI, AIOps becomes a self-learning system that continuously optimizes itself. Skilled AI developersare required to equip IT structures with tools tailored precisely to specific requirements.
The Key Aspects Of AI integration Are:
- Data Analysis: Large volumes of operational data from various sources like logs, metrics, and events are analyzed, and AI models identify patterns and anomalies in the data to indicate potential issues.
- Anomaly Detection: AI-powered IT structures can recognize deviations from normal operational patterns and identify anomalies or exceptional activities.
- Automation: AI is used to automate routine tasks and decisions in IT operations, from resource scaling to executing maintenance tasks.
- Capacity Planning and Optimization: AI monitors resource utilization and forecasts future capacity requirements, optimizing resource allocation and avoiding bottlenecks.
- Prevention and Forecasting: By training ML models on historical data, AIOps can predict future trends and issues, allowing timely preventive measures to prevent possible failures or disruptions.
You can find more information about the potential of AI here.
How Does AIOps Work?
The functioning of Artificial Intelligence for IT Operations can be outlined through the following steps:
- Data Collection and Aggregation: AIOps begins by collecting extensive datasets from various parts of the IT infrastructure, such as logs, metrics, events, and user activities.
- Data Processing and Preparation: The collected data is cleaned, transformed, and prepared for analysis, including removing duplicates, merging data sources, and aggregating metrics.
- Pattern and Anomaly Detection: AI models and ML algorithms are used to identify patterns and anomalies within the data, detecting abnormal behavior and anomalies that may indicate problems or disruptions.
- Correlation and Root Cause Analysis: AIOps platforms can correlate various events and data to identify potential causes for anomalies.
- Automation: Based on the detected patterns, Artificial Intelligence for IT Operations can trigger automated responses or actions, such as automatic resource scaling, performing maintenance tasks, or initiating troubleshooting measures.
- Reporting and Visualization: The insights gained can be presented in clear dashboards and reports.
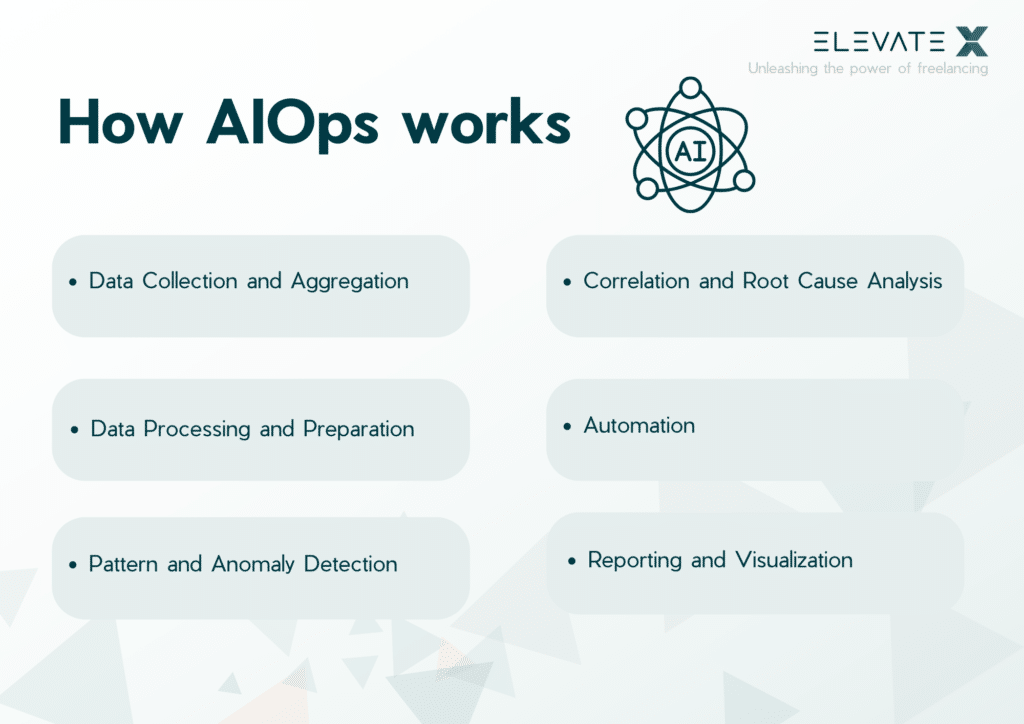
What Is The Difference Between Artificial Intelligence For IT Operations And MLOps?
AIOps and MLOps define two areas of the same process: while Artificial Intelligence for IT Operations focuses on the operation of IT systems, MLOps – Machine Learning Operations – ensures the effective use of ML models in business operations. The scope of MLOps includes managing machine learning models from development to deployment and maintenance in production environments. Machine Learning Operations are designed to bridge the gap between data scientists who develop models and the IT teams responsible for putting these models into operation. They encompass specific processes, tools, and methods that ensure and facilitate the profitable integration, deployment, and monitoring of ML models within your company. Detailed explanations about MLOps can be found here.
The following tools and platforms are characteristic of MLOps:
- Kubeflow supports the deployment, management, and scaling of ML models in Kubernetes clusters.
- MLflow: simplifies the management of ML models from prototype development to deployment. MLflow enables experiment tracking, model storage, and deployment.
- Data Version Control – DVC: allows tracking of data, models, and experiments, supporting the repeatability of ML workflows.
- TensorFlow Extended – TFX: creates production pipelines for ML models. The platform includes tools for data validation, model analysis, prediction, and evaluation pipelines.
However, efficient utilization of MLOps is only possible with the involvement of an experienced specialist. Here, you can find skilled machine learning engineers who are well-versed in this field.
What Is The Difference Between AIOps And DevOps?
The focus of AIOps is on the use of AI and ML in IT operations. On the other hand, DevOps describes the methodology of collaboration and coordination between software development (Development) and operations (IT Operations). The goal of DevOps is to accelerate the delivery of business-ready software, improve its quality, and foster collaboration between different teams. DevOps encompasses practices like continuous integration (CI) and continuous deployment (CD), automation of deployment processes, and the use of infrastructure-as-code (IaC).

Are you looking for a Prompt Engineer?
What Are Some Use Cases Of AIOps?
The IT research institute Gartner defines five primary application segments for AIOps. The first and fundamental one is optimizing Big Data management. Large amounts of operational and performance data from various sources such as servers, networks, applications, and sensors are analyzed and prepared concerning volume, variability, variety, and velocity. Additionally, AIOps can use ML models to make predictions about future trends, data volumes, or performance metrics, which can be helpful in optimizing resources and capacity planning. The Big Data engineer is responsible for implementing appropriate tools and ensuring smooth interaction with other components of the IT infrastructure.
Other Application Areas Include:
- Real-time performance analysis: AIOps can provide analysis functions to process large data streams in real-time and immediately respond to deviations or issues. This is particularly crucial for timely action during critical events. The immediate identification and resolution of faulty or incomplete data guarantee reliable analyses based on high-quality data.
- Anomaly detection: AIOps identifies anomalies and unusual activities within large data sets using algorithms. The model enables early detection of security breaches, system failures, or performance problems. Outlier detection identifies data outliers that significantly deviate from “normal” data and may indicate potential issues.
- Automation: AIOps’ automated data segmentation can help automatically divide large data sets into relevant segments for targeted analysis and reporting. This includes cloud monitoring to monitor available cloud infrastructure, storage monitoring, and virtualization monitoring. Automated scaling of Big Data platforms by AIOps enables resource provisioning according to current requirements.
- IT service and maintenance management: AIOps provides support in identifying the root causes of errors by recognizing patterns or dependencies between various data points. Data processing pipelines in Big Data environments are monitored to ensure smooth data flow.
Are you enticed to participate as an IT professional in tackling such demanding tasks? Then make use of our extensive network for continuously new jobs and a secure income.
What AIOps Tools Are There?
Versatile AIOps tools optimize IT operations through the use of AI and ML. Here are some of the most common ones:
- Dynatrace: A platform for IT management that leverages AI to monitor and optimize the performance of applications, infrastructure, and user experiences.
- Splunk IT Service Intelligence: A tool for event correlation and analysis that uses AI and ML to detect and resolve IT operation issues in real-time.
- Moogsoft: Another event correlation tool that uses AI to identify, prioritize, and trigger alerts for events as needed.
- AppDynamics: The platform provides AI-powered insights into IT applications and their infrastructure.
- ServiceNow ITOM Predictive AIOps: A tool for AI-driven predictions and automation of IT operations.
Other tools that can be used depending on the specific IT infrastructure include: New Relic, PagerDuty, ScienceLogic, IBM Watson AIOps, and OpsRamp.
What Are The Benefits Of AIOps For A Company?
From the broad range of AIOps benefits, here are the key ones highlighted:
- Proactive management by detecting and automatically resolving issues and anomalies in real-time, resulting in reduced disruptions or downtime and improved service levels.
- More efficient use of a company’s IT resources through automatic scaling and redistribution according to current demands. AIOps platforms can seamlessly integrate into complex IT infrastructures, allowing flexible adaptation to changing requirements.
- Improved service quality through continuous monitoring and analysis of service performance data.
- Automated processes: AIOps can automate repetitive tasks and manual processes, such as event monitoring, log analysis, and report generation.
- Data-driven decision-making: Artificial Intelligence for IT Operations provides comprehensive insights into IT operations based on analyses of large data sets. This enables informed and data-driven decisions that make the company more effective and agile.
- Time and cost savings through process automation, more efficient resource utilization, and rapid problem identification.
If you want to harness these benefits for your company, an IT project manager is ready to assist you with advice and support.
What Are The Disadvantages Of Artificial Intelligence For IT Operations?
The implementation of AIOps can be very complex and time-consuming, requiring changes to existing IT systems, data sources, and processes. Possible issues related to this are explained in a report by the Gartner Institute, which you can find information about on this webpage. Additional training may be necessary. A potential drawback could be that decisions made by AIOps models are sometimes difficult to understand, especially when complex AI algorithms are involved. Human supervision of AI decisions is essential to avoid misinterpretations. A reliable data privacy protection is of particular importance.
AIOps stands for Artificial Intelligence for IT Operations and refers to the use of AI and ML to optimize and automate IT operational processes and activities. It includes the analysis of operational data, anomaly detection, automated responses, and improvement of service quality.
Examples of AIOps tools are AI-powered platforms like Dynatrace, Splunk IT Service Intelligence, AppDynamics, Moogsoft, and New Relic.
AIOps is used in IT organizations to optimize the operation of IT infrastructures, detect disruptions early, and enable automated measures to improve service quality. It finds widespread application in various industries such as financial services, healthcare, e-commerce, and telecommunications.